Virtual Data Room (VDR) replaces Physical Data Rooms (2002-2022)
20 years ago, the M&A Due Diligence (DD) process changed fundamentally with the introduction of Virtual Data Rooms. Before then, DD was conducted by designating a separate physical room at the seller’s office, where physical copies of all company documentation pertinent to the DD were made available. It’s hard to believe now that it used to work that way, and hard to imagine how inefficient this process was. The buyers, or their advisors, would have to visit the seller’s office to investigate the documentation. Cross-border deals were therefore not straightforward, obviously.
VDRs made the process much more efficient, and more effective, as data was made accessible remotely, securely, and instantly. Imprima was a pioneer at the time, as the first Europe-based VDR supplier.
Next Generation Smart VDR replaces Standard VDRs (2022 & beyond)
Fast forward 20 years to today and again a paradigm shift is taking place. Like the fundamental change to the DD process that occurred by the introduction of the first VDR, this new shift will have great impact and be permanent. And again, Imprima is at the forefront, the pioneer, as the first VDR supplier to embark on this path, and clearly ahead of its competition.
The current paradigm change has to do with the distinction between data and information, and what humans like to do, and what not – and what they are good at, and again what not.
The problem – finding the information
While VDRs allow the data to be accessed efficiently and remotely, it is still hard and very laborious to find the information in the data. The information you need is often buried deep down in the documents to be analyzed. And there are a lot of documents to deal with in any DD process. The most valuable firms being sold have large groups of customers. As a result, such firms will have an abundance of commercial agreements, lease agreements, license agreements etc. These agreements are often similar, but not the same. If they were exactly the same, retrieving information from them (e.g. the lease term for a Real Estate deal, the license fee when software companies are involved, etc.) would be easy: a simple search would do the job. It is not at all easy, however, if they are not the same. Even if there are only slight differences as to how something is worded, simple searches won’t work.
Therefore, when preparing and executing DD and VDD, it is done manually, by reading the documents. This is obviously very repetitive work, and hence very tedious for the analysts and lawyers that must plow through all that data. Young and bright people, analysts, lawyers and paralegals alike, are being tasked to analyze documents that are very similar, holding the same kind of information, again and again, document after document, deal after deal, over and over again. Not a good situation obviously. Not efficient at all, and not at all motivating and rewarding work. Not good for staff retention either. In other words, a major problem for any party involved in DD.
The solution – Machine Learning
That is where Machine Learning comes in. At Imprima, we developed Machine-Learning technology that is able to recognize the context of the terms that you want to find (e.g. start date, end date, contract parties, etc.), rather than just the terms themselves. It can do this in multiple languages, and “learns” and predicts across multiple languages as well. As a result, it can find what you are looking for in texts that are semantically the same, but not verbatim the same. More detail on this can be found here.
To show how that works in practice, let’s discuss an important use case of Machine Learning in a DD context. DD reports for Vendor DD. We will use documentation for a Real-Estate transaction as an example.
Use case: Due Diligence (“Red Flag”) Reports
Understanding the value and risk of a deal, and verifying facts is a crucial element of a deal phase. To achieve that, advisors review relevant documentation and produce a DD report (or “red flag report”) for their customers.
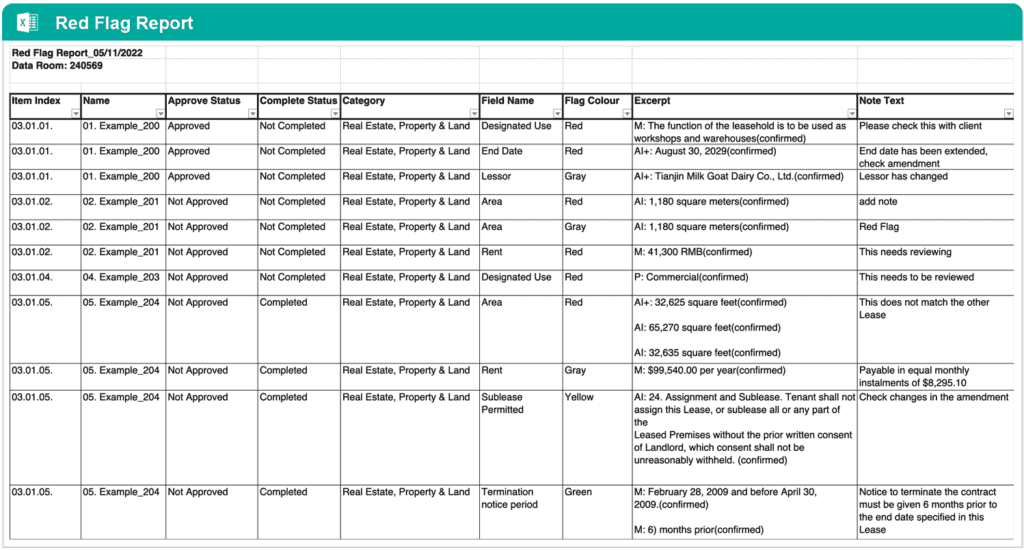
Obviously, due to the exponential increase of data in the past few years, and the high time pressure when conducting DD, reviewing all the documentation thoroughly has become a daunting task. Making reports and analyzing large numbers of documents manually is cumbersome and error prone, often involving copying information from documents into a different word or excel report.
How improve that process
Imprima’s DD toolkit uses unique and innovative Machine Learning technology to assist with this process. Users can specify fields that they want to review, such as contract term, termination rights and change of control, and build a template for the review. During the review, the algorithm learns from the user’s interaction with the documents and assists by identifying semantically similar clauses or datapoints in other documents in the dataset. Using this combination of machine learning and human knowledge, the user is quickly able to acquire information on a whole portfolio of contracts. With information being readily available and all in one place, the assessment of risk and value is made much more efficient.
Key to the efficiency of the process is how much information can be automatically extracted.
We tested the Machine Learning on the documentation of a Real Estate transaction, which consisted of Dutch documents.
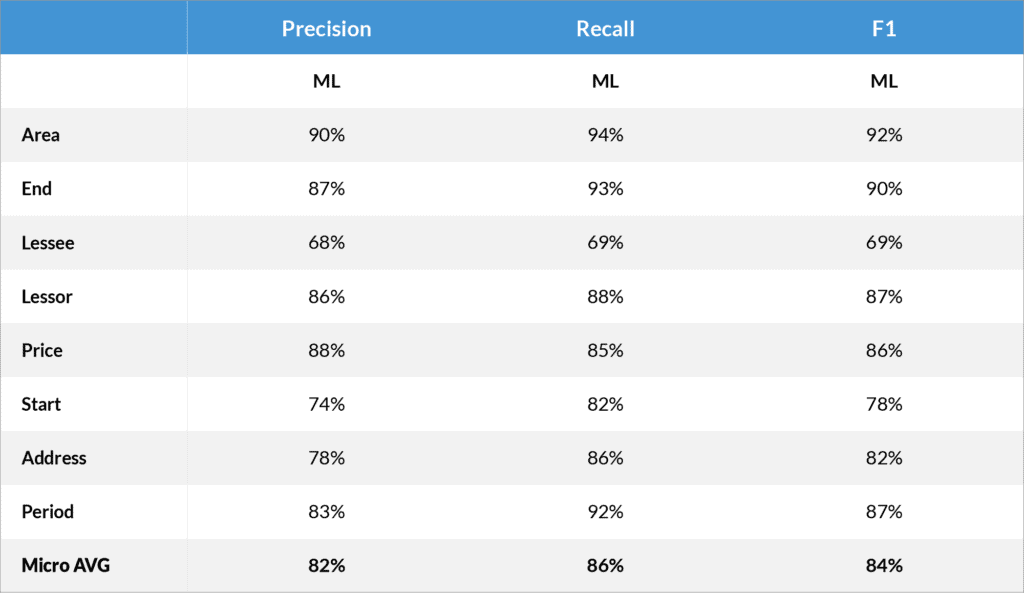
In the Imprima Smart VDR toolkit, the extracted information is automatically fed into a comprehensive report of all documents to be analysed:
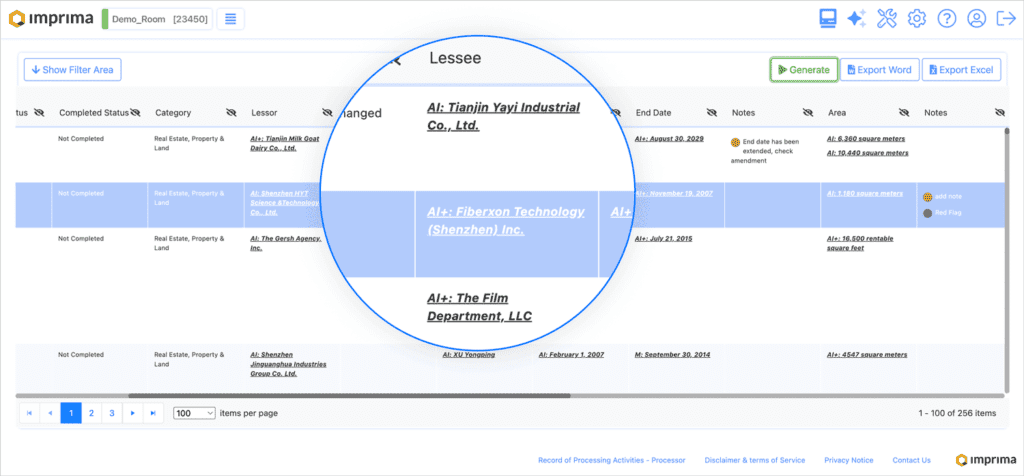
Users are now able to see, at a glance, which data points have – and which have not – been retrieved by the AI. From the report, they can open the documents to review and the extracted datapoints for further review and approval, or drill down into a (missing) data point to enter, review or edit it.
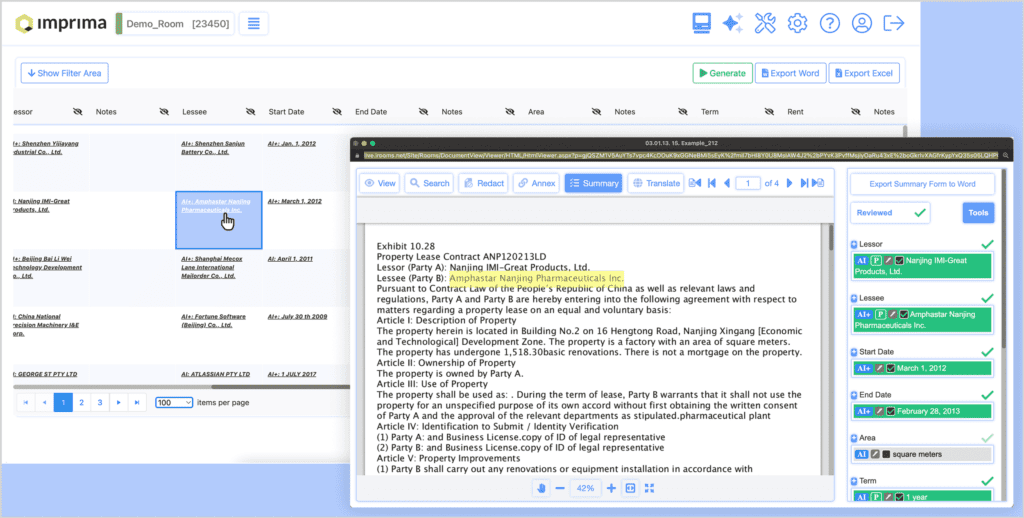
Then, in a final step, the red flag report can be generated, showing key data for only those documents where red flags are detected, and again allowing users and their clients to conveniently drill down into the report:
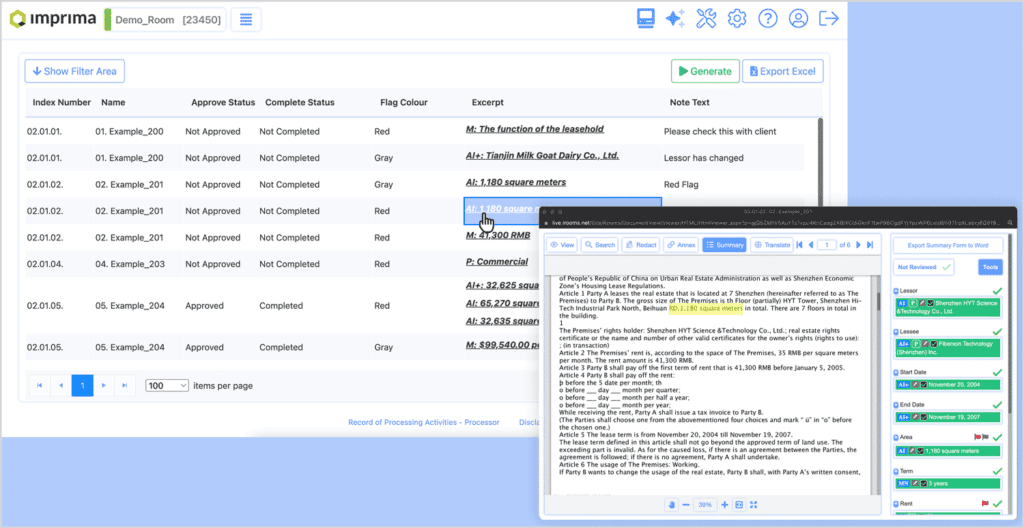
It goes without saying that this approach saves an enormous amount of time. Not only is the majority of data points automatically retrieved (86% as in the test results above), but it has also become very easy to check the retrieved data points, enter missing ones, and approve the results.
At Imprima, we are dedicated to improving the DD process and making your life easier while we are at it.
Get in touch to make our technology work for you: Contact Us