Gimmick or Does it Add Value?
See Imprima AI in action here
Developments in legal AI are being followed closely by all major law firms. For these law firms, the application of AI for the analysis of legal documents holds tremendous potential and could potentially be a threat if not adopted
in a timely fashion.
However, most law firms that we have spoken with have not yet fully embraced it, are not – in practice – really using it, or are at best in the evaluation mode. There is a lot of potential, but it would appear that ‘legal AI is at the “frothy” part of the hype cycle’, as one lawyer aptly stated on his blog. Why is that? There are various reasons for this.
Key factors behind slower adoption rates of automated solutions:
Too much data and too much effort required
Large amounts of data (legal documents) are needed to “train” the commonly used AI algorithms. However, the data is generally owned by third parties, and not available for that purpose. Moreover, these AI tools cannot be used without lawyers investing large amounts of time and effort to get the technology to work for them, in delivering results: It is frequently
the case that algorithms need to be trained by “labelling” large amounts of documents, which can only be done by people with sufficient expertise: i.e. the lawyers themselves.
We believe, however, that these issues can be overcome when the appropriate AI algorithms are utilised and appropriate workflows implemented. We will discuss this further in a future post.
It doesn’t do what it needs to do
First of all, as we understand from our conversations with a multitude of law firms, current AI technology on the market is not solving their real day-to-day problems. For instance, a common application of AI is to find the needle in the haystack, i.e. find that one provision that represents a critical risk hidden in a large number, thousands, or tens of thousands, of documents. While something like that may be a valid use case for e-discovery in litigation, for instance, it is not a valid use case in the typical M&A transaction.
Underestimation of time & costs involved in the VDR preparation process
In the average Virtual Data Room (VDR) hundreds – not tens of thousands – of legal documents are present. As such the ultimate perception may be that the process is efficient i.e. having to browse through a data room of that size is really not that time-consuming. However, in practice, the real challenge is to get the data into the data room, when many more documents – often ten-fold or more – have to be searched and sorted, with older and
duplicate versions removed and then organised into a VDRindex.
Reliability concerns
Significant time savings and efficiencies can be achieved by automating this process. But that only works if it can be done reliably: In many SPA’s, anything that is contained in the documents presented in the VDRare deemed to be disclosed. In any case, the selling party wants to be sure that all relevant documentation has been found and uploaded to the VDR.
And that is a pre-condition for any legal AI use case to work: it needs to be sufficiently accurate, and sufficiently reliable. In the remainder of this post, we will focus on the reliability of AI for Mergers and Acquisitions Due Diligence.
How to measure reliability? How reliable is reliable enough?
First of all, we have to ask ourselves how reliability should be measured. In our post of February 2018 (click to read), we argued that the key to making AI work in legal due diligence is optimal accuracy as measured by the so-called “recall”.
The other measure of accuracy in AI, “precision”, is of less importance. Why is that? What are recall and precision precisely?
To start with the latter question:
• Recall is a measure of the number of “false negatives”, documents (or information items) that have failed to be identified, that have been missed by the query.
• Precision is a measure of the number of “false positives”, documents that are presented to the user as matching the user’s query, but really don’t.
So why is it key to get an optimal recall, even at the cost of some precision? That can be illustrated by the chart below. In conclusion, recall measures the reliability of your result; whether you can be sure that you will find all the documents that you need to find.
Automated Information Retrieval (AIR) vs. Manual Search example
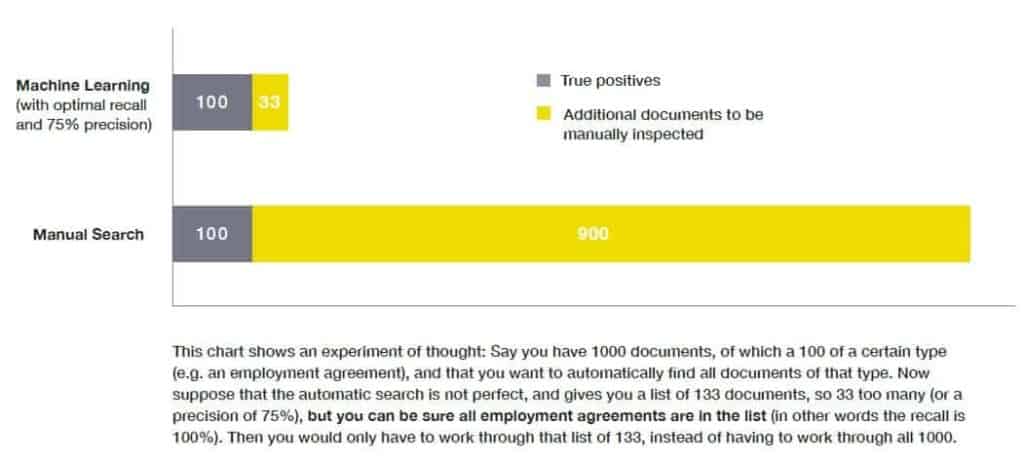
But how sure is sure? 100% sure? Unfortunately, that does not happen in the real world. Then again, does manually searching through all documents ensure 100% recall? Though it is difficult for humans to admit that they
make mistakes, they do. In fact, a paper by Grossman & Cormack (2011) argues that human recall is not likely to be ever even close to 100%, and shows that in a variety of tests human recall ranges from 50% – 85%.
It is more a psychological issue than a scientific issue or a business issue: Humans rather accept mistakes by people than mistakes by a “machine”, even if mistakes by a machine are much less likely to happen. This phenomenon has been identified as “algorithm aversion” by Dietvorst et al, 2014, and there are many examples of this in daily life. For instance, the one fatal accident with a driverless car makes all the front pages (there have been 4 in total so far), while fatal accidents with cars happen every day (40.100 in 2017 in the USA alone).
Nevertheless, the truth of the matter is that if we can reduce human error in a significant way, we are better off, in reality, from a business point of view. This perception issue will change over time, once people start to realise that we need to look at reliability in an objective, not subjective, way.
Reducing the risk of error in real terms is what we are focusing on at Imprima. In designing Imprima’s Virtual Data Room, platform, it always has been a key objective to increase security by reducing the likelihood of human error (as most security breaches are the result of human error, not penetration by a third party). Now we are using AI to even further reduce the likelihood of human error.
Reliability results
What can be attained in terms of reliability? In the following experiment, we used Imprima’s proprietary Machine Learning to find [4] categories of agreements (consulting agreements, employment agreements, license agreements, and loan agreements) from a pool of 4,631 legal documents.
The results were as follows:
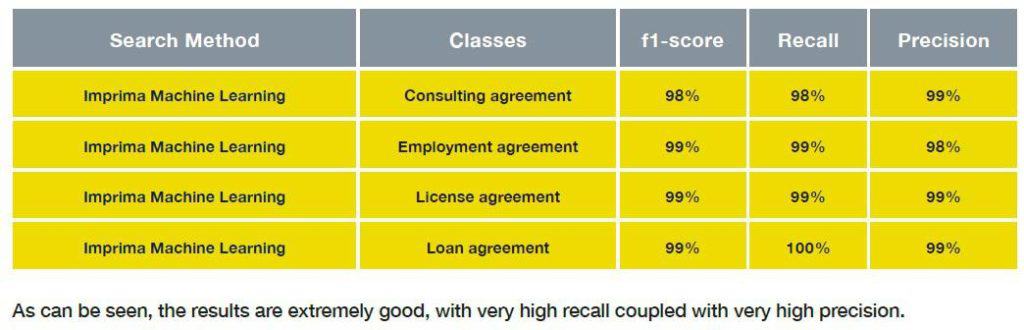
- 98%-100% precision, or only a few percent “too many” documents were identified. For instance, in case of the loan agreements, this means that the user when evaluation the 999 automatically selected agreements, will have to discard only 9 .That is a whole lot less work than having to manually search through all the 4,631 documents.
- The most important finding is, however, that a very high recall was obtained, 98%-100% as well, so only very few agreements searched for were missed. Would a human ever be able to do better, even given unlimited time (which will never happen in practice)?
Conclusion
In conclusion, we believe that we can attain sufficiently reliable results, so the precondition to making AI work in legal DD can be satisfied. This precondition is particularly of importance in the “pre-VDR” process (where documents have to be searched, sorted, older versions removed, and then organised into a VDR index), where one wants to be sure that no documents have been missed.
We have implemented the pre-VDR process in our recently released Imprima AI for M&A Due Diligence product, along with many other workflows, including document classification, information retrieval to create document summaries, legal clause extraction, and detection of legal red flags in documents.
Citations
- https://in-houseblog.practicallaw.com/what-has-ai-ever-done-for-us/
- https://en.wikipedia.org/wiki/List_of_autonomous_car_fatalities
- https://www.usatoday.com/story/money/cars/2018/02/15/national-safety-council-traffic-deaths/340012002
References
Maura R. Grossman & Gordon V. Cormack, Technology-Assisted Review in E-Discovery Can Be More Effective and More Efficient Than Exhaustive Manual Review, XVII RICH. J.L. & TECH. 11 (2011), https://jolt.richmond.edu/v17i3/article11.pdf.
Dietvorst, Berkeley and Simmons, Joseph P. and Massey, Cade, Algorithm Aversion: People Erroneously Avoid Algorithms after Seeing Them Err (July 6, 2014). Forthcoming in Journal of Experimental Psychology: General. Available at SSRN: https://ssrn.com/abstract=2466040 or https://dx.doi.org/10.2139/ssrn.2466040